USING PREDICTIVE ANALYTICS TO IMPROVE SURVEILLANCE OF HEAT-RELATED ILLNESS DURING MILITARY TRAINING
DOI:
https://doi.org/10.55374/jseamed.v8.189Keywords:
heat-related illnesses, heat stroke, machine learning, military, predictionAbstract
Background: Heat-related illnesses are a critical concern for military personnel, especially those unfamiliar with hot climate regions. The Royal Thai Army (RTA) implements a 10-week military training program encompassing four phases: (1) Heat acclimatization training, (2) Combat fundamentals and unarmed combat training, (3) Armed combat training and tactical training, and (4) Field training exercise and evaluations.
Objective: This study aimed to conduct a predictive analysis of heat-related illnesses to enhance prevention programs.
Methods: The study utilized secondary data from the RTA Medical Department, incorporating variables such as age, occupation, education, underlying diseases, smoking and alcohol consumption, sleep duration, exercise, medication history, body mass index (BMI), weight loss, body temperature, dark urine, heat rash, and environmental humidity. Multiple machine learning algorithms were employed to develop predictive models.
Results: The samples comprised 809 male recruits (103,051 encounters) with an average age of 22. Approximately 12% of the recruits had a BMI ≥30 kg/m2, while nearly 70% and 90% reported tobacco use and alcohol consumption in the past 12 months, respectively. Among the recruits, 16% reported substance use within the preceding 30 days. The eXtreme Gradient Boosting (XGB) model achieved 91% accuracy in predicting heat-related illnesses before Phase 2. The top five predictive variables were Lopburi Province (central region), Songkhla Province (southern region), and Bangkok (capital city), sleep duration before joining military training (hours), and age (years).
Conclusion: This study, which applied machine learning techniques to predict heat-related illnesses among Thai recruits, can potentially impact the health and training of military personnel. The comparative analysis of various algorithms identified the XGB model as the optimal performer in predicting heat-related illnesses during the combat fundamentals and unarmed combat training phase. However, it is essential to note that further study is needed to enhance the applicability of our predictive model, which includes expanding its use to new cohorts of Thai conscripts, underscoring our research’s ongoing nature.
Metrics
References
Atha WF. Heat-Related Illness. Emerg Med Clin North Am 2013; 31: 1097-108. DOI: https://doi.org/10.1016/j.emc.2013.07.012
Bouchama A, Knochel JP. Heat Stroke. N Engl J Med 2002; 346: 1978-88. DOI: https://doi.org/10.1056/NEJMra011089
Epstein Y, Roberts WO. The pathophysiology of heat stroke: an integrative view of the final common pathway. Scand J Med Sci Sports 2011; 21: 742-8. DOI: https://doi.org/10.1111/j.1600-0838.2011.01333.x
Yamamoto T, Fujita M, Oda Y, Todani M, Hifumi T, Kondo Y, et al. Evaluation of a novel classification of heat-related illnesses: A multicentre observational study (heat stroke study 2012). Int J Environ Res Public Health 2018; 15: 1962. DOI: https://doi.org/10.3390/ijerph15091962
Bouchama A. Prognostic factors in heat wave–related deaths, a meta-analysis. Arch Intern Med 2007; 167: 2170. DOI: https://doi.org/10.1001/archinte.167.20.ira70009
People’s Liberation Army Professional Committee of Critical Care Medicine. Expert consensus on standardized diagnosis and treatment for heat stroke. Mil Med Res 2016; 3: 1. DOI: https://doi.org/10.1186/s40779-015-0056-z
Casa DJ, Armstrong LE, Ganio MS, Yeargin SW. Exertional heat stroke in competitive athletes: Curr Sports Med Rep 2005; 4: 309–17. DOI: https://doi.org/10.1097/01.CSMR.0000306292.64954.da
Williams V, Oh G-T. Update: heat illness, active component, US Armed Forces, 2021. MSMR 2022; 29: 8–14.
Kiatkitroj K, Arphorn S, Tangtong C, Maruo SJ, Ishimaru T. Risk factors associated with heat-related illness among sugarcane farmers in Thailand. Ind Health 2021; 60: 447–58. DOI: https://doi.org/10.2486/indhealth.2021-0161
Gardner JW, Kark JA, Karnei K, Sanborn JS, Gastaldo E, Burr P, et al. Risk factors predicting exertional heat illness in male Marine Corps recruits. Med Sci Sports Exerc 1996; 28: 939-44. DOI: https://doi.org/10.1097/00005768-199608000-00001
Kim S-H, Jo S-N, Myung H-N, Jang J-Y. The effect of pre-existing medical conditions on heat stroke during hot weather in South Korea. Environ Res 2014; 133: 246-52. DOI: https://doi.org/10.1016/j.envres.2014.06.003
Sawka MN, Gonzalez RR, Pandolf KB. Effects of sleep deprivation on thermoregulation during exercise. Am J Physiol 1984; 246: R72-77. DOI: https://doi.org/10.1152/ajpregu.1984.246.1.R72
Martinez M, Devenport L, Saussy J, Martinez J. Drug-associated heat stroke. South Med J 2002; 95: 799–802. DOI: https://doi.org/10.1097/00007611-200295080-00006
Coris EE, Ramirez AM, Van Durme DJ. Heat illness in athletes: the dangerous combination of heat, humidity and exercise. Sports Med Auckl NZ 2004; 34: 9-16. DOI: https://doi.org/10.2165/00007256-200434010-00002
Cleary M. Predisposing risk factors on susceptibility to exertional heat illness: clinical decision-making considerations. J Sport Rehabil 2007; 16: 204-14. DOI: https://doi.org/10.1123/jsr.16.3.204
Bedno SA, Urban N, Boivin MR, Cowan DN. Fitness, obesity and risk of heat illness among army trainees. Occup Med Oxf Engl 2014; 64: 461-7. DOI: https://doi.org/10.1093/occmed/kqu062
American College of Sports Medicine, Armstrong LE, Casa DJ, Millard-Stafford M, Moran DS, Pyne SW, et al. American College of Sports Medicine position stand. Exertional heat illness during training and competition. Med Sci Sports Exerc 2007; 39: 556–72. DOI: https://doi.org/10.1249/MSS.0b013e31802fa199
Epstein Y, Druyan A, Heled Y. Heat injury prevention--a military perspective. J Strength Cond Res 2012; 26 Suppl 2: S82-86. DOI: https://doi.org/10.1519/JSC.0b013e31825cec4a
Nutong R, Mungthin M, Hatthachote P, Ukritchon S, Imjaijit W, Tengtrakulcharoen P, et al. Personal risk factors associated with heat-related illness among new conscripts undergoing basic training in Thailand. PloS One 2018; 13: e0203428. DOI: https://doi.org/10.1371/journal.pone.0203428
Jamei M, Nisnevich A, Wetchler E, Sudat S, Liu E. Predicting all-cause risk of 30-day hospital readmission using artificial neural networks. PLOS ONE 2017; 12: e0181173. DOI: https://doi.org/10.1371/journal.pone.0181173
Morgan DJ, Bame B, Zimand P, Dooley P, Thom KA, Harris AD, et al. Assessment of machine learning vs standard prediction rules for predicting hospital readmissions. JAMA Netw Open 2019; 2: e190348. DOI: https://doi.org/10.1001/jamanetworkopen.2019.0348
Narla A, Kuprel B, Sarin K, Novoa R, Ko J. Automated classification of skin lesions: From pixels to practice. J Invest Dermatol 2018; 138: 2108–10. DOI: https://doi.org/10.1016/j.jid.2018.06.175
Gulshan V, Peng L, Coram M, Stumpe MC, Wu D, Narayanaswamy A, et al. Development and validation of a deep learning algorithm for detection of diabetic retinopathy in retinal fundus photographs. JAMA 2016;3 16: 2402– 10. DOI: https://doi.org/10.1001/jama.2016.17216
Nelder JA, Wedderburn RWM. Generalized linear models. J R Stat Soc Ser Gen 1972; 135: 370. DOI: https://doi.org/10.2307/2344614
Zhang Z. Introduction to machine learning: k-nearest neighbors. Ann Transl Med 2016; 4:218. DOI: https://doi.org/10.21037/atm.2016.03.37
Breiman L. Random Forests. Mach Learn 2001; 45: 5–32. DOI: https://doi.org/10.1023/A:1010933404324
Chen T, Guestrin C. XGBoost: A Scalable Tree Boosting System. Proc. 22nd ACM SIGKDD Int. Conf. Knowl. Discov. Data Min., San Francisco California USA: ACM; 2016, p. 785–94. DOI: https://doi.org/10.1145/2939672.2939785
Zurada JM. Introduction to artificial neural systems. West Publishing Company, Newyork 1992, 1st edition
O’Shea K, Nash R. An introduction to convolutional neural networks 2015. Retrieved from arXiv:1511.08458.
Chawla NV, Bowyer KW, Hall LO, Kegelmeyer WP. SMOTE: Synthetic minority over-sampling technique. J Artif Intell Res 2002;16:321–57. DOI: https://doi.org/10.1613/jair.953
Kuhn M. Building predictive models in R using the caret package. J Stat Softw 2008; 28: 1–26. DOI: https://doi.org/10.18637/jss.v028.i05
Kalinowski T, Falbel D, Allaire JJ, Chollet F, RStudio, Google T, [ctb Y, cph, Bijl WVD, Studer M, Keydana S, 2023. “Keras: R Interface to ’Keras’. DOI: https://doi.org/10.32614/CRAN.package.keras3
Krittanawong C, Johnson KW, Rosenson RS, Wang Z, Aydar M, Baber U, et al. Deep learning for cardiovascular medicine: a practical primer. Eur Heart J 2019; 40: 2058-73. DOI: https://doi.org/10.1093/eurheartj/ehz056
Liaw A, Wiener M. Classification and regression by Random Forest. R News 2002; 2: 18–22.
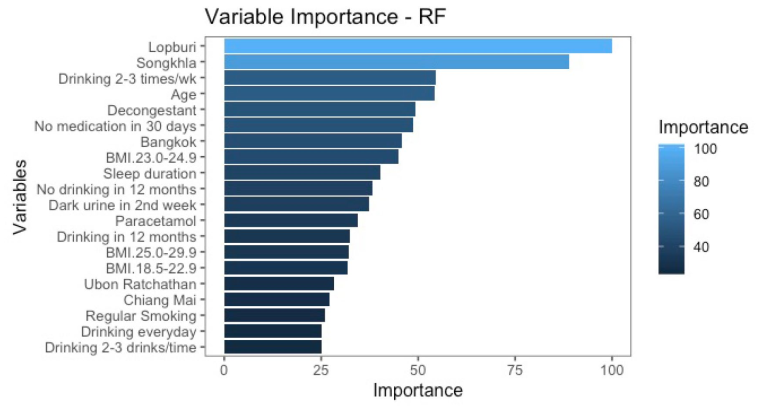
Downloads
Published
How to Cite
Issue
Section
License
Copyright (c) 2024 Journal of Southeast Asian Medical Research

This work is licensed under a Creative Commons Attribution-NonCommercial-NoDerivatives 4.0 International License.
The Journal of Southeast Asian Medical Research will hold the copyright to all published articles. The publisher's production department handles copyright forms once a manuscript is accepted and scheduled for publication.